Retailer Saves Millions and Improves Comfort Using Agentic AI
Company: National Small-Box Discount Retail Store
Result: 503% Average Monthly ROI | $2.5 Million Annual Savings
Scale: 1,800+ Retail Stores. Over 5,000 Rooftop Units Nationwide
Overview
It started with a “bake-off” for 90 days to evaluate the effectiveness of AI: twenty stores managed by the incumbent BMS vendor’s “AI demand management” partner, twenty stores managed by facil.ai’s AI-agents. facil.ai secured the contract by saving 19% more energy than the incumbent.
Facil.ai was implemented in the entire portfolio in June 2022, after which the retailers saw average monthly energy savings of 10% and a monthly average of $250,000 cost savings.
This retailer has seen tremendous growth over the past few years and has more than doubled their footprint since implementing facil.ai. New sites are on-boarded automatically to the facil.ai platform within minutes, immediately saving energy.
Due to the tremendous savings from the Rooftop Unit Optimization AI-agents, the facil.ai contract was extended for another three years. Next steps for this retailer is to use facil.ai for preventative maintenance analysis.
Key Results
Saving Energy
19.5 Million kWh saved annually
The portfolio reduced their energy usage by over 19.5 million kWh’s. That is enough to power a small data center for a year.
Ongoing Payback
503% monthly ROI
The monthly savings overshadowed the ongoing SaaS fee. The company saw an average monthly ROI of 503%.
Validated Savings
$2.5Million Saved
The company is saving roughly $2.5 Million annually and new sites are automatically integrated in minutes.
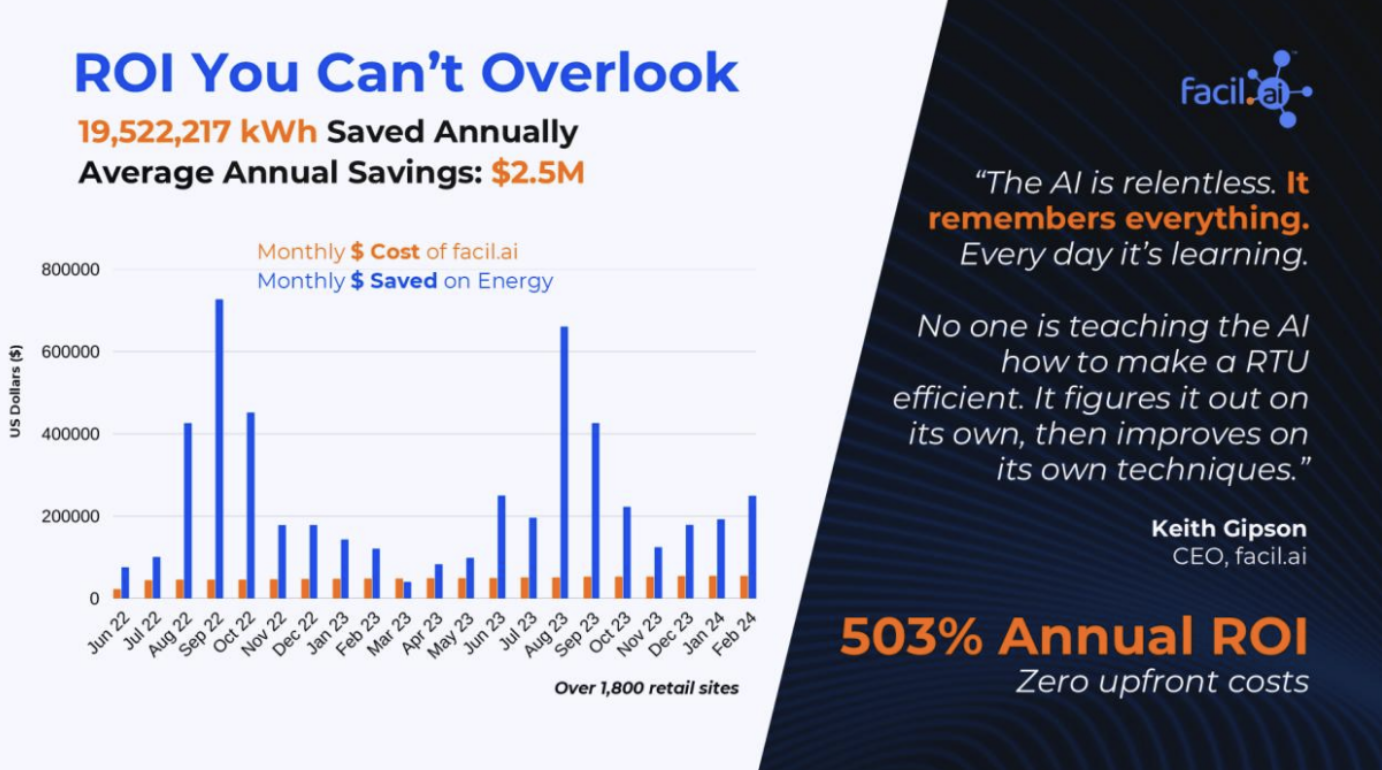
Additional Results
- 30% enhancement in comfort (more zones at corporate comfort settings)
- 40% reduction in maintenance calls, alarms, and truck rolls
System Details
- Cloud to cloud engagement with incumbent vendor
- Smart Thermostat BMS Company
- API integration
- No on-site hardware from facil.ai
Testimonial
“Results from the pilot were astounding. The facil.ai team brought benefit after benefit. After awhile, I just told them, ‘You HAD me at 19%! (energy savings). If you keep saving me money like this, we are never going to cancel.’”
--VP of Procurement
Previous Energy Saving Attempts
Previously they were doing manual oversight (2 people) plus an outside consultant, manning front ends for 1,000 stores in an effort to manage energy and maintenance. The retailer was focused on reacting to energy, comfort, and maintenance issues. There were no proactive energy conservation measures.
By optimizing the performance and efficiency of the rooftop units, the retailer has 30% better comfort settings and has been able to reduce their alarms and truck rolls by 40% (a positive side effect of efficiency).
AI Strategy
The AI uses Adaptive Real-time Inference Learning. This is the AI-agents’ ability to Observe real-time data, Plan the best strategy, Act by sending a command to the BMS, then Learn from the resulting outputs. The AI-agents are continually adapting and learning, training themselves based on previous adjustments to improve efficiency.
Initially, the system starts in learning mode. This is the first step, where the AI agents safely observe the controls and zone temperatures for each unit/zone and learns over time how best to dynamically configure and adjust each unit precisely to increase energy efficiency. Within a couple of weeks, the AI agents have learned how to optimize each RTU and start making micro adjustments to reduce energy use while maintaining precise comfort.
The AI uses the concept of building as a battery (BaaB). The AI builds a unique model for each of the zones and the agents optimizes each zone like a snowflake. There is one model per zone, not per building. The AI optimizes each unique zone relentlessly.